Data Glance
- 100 GB of data
- 20K-30K orders/month
- 30 customers using dashboards
Customer’s Response
“Firebolt allowed us to build the product we always envisioned building, fully accompanied with robust features. Firebolt opened up the aperture of our product's desired features and capabilities.” - Troy Bolus, Co-Founder at OmniPanel
The Company
OmniPanel provides e-commerce insights for B2B and B2C companies by integrating data from companies like Shopify, Returnly, and Sendgrid. Their software connects the dots between customers, orders, shipping, returns, marketing, support, and more to supercharge customer insights reporting cross-functional work.
The Challenge: Growing Customer Data and Complex Analytics
While OmniPanel was building out a technology stack, they began to identify that the data layer would not scale to meet the needs of their customers. They searched for a solution that would allow them to scale to support their growing customer data volumes and complexity of analytics, without requiring a large amount of overhead to deploy.
The Solution: 15x Faster Performance
After evaluating a number of solutions, Firebolt stood out as the leader in both current performance and projected growth. Firebolt’s performance was 15x faster than the current Postgres database, with an average query time of less than 500 milliseconds.
OmniPanel was able to increase the performance even further by converting their data model from a 21-table 3rd normal form structure into a denormalized 3 table structure. This change allowed for taking advantage of Firebolt’s aggregating indexes, and further reduced the complexity and speed of the queries.
With customer success as the highest priority, the Firebolt team partnered with the OmniPanel team to provide best practices and recommended schema and query changes to optimize the database to scale as OmniPanel grows.
Run queries at any scale in sub seconds:

Data Model
The original data model for OmniPanel was a normalized data model consisting of 21 tables. This type of structure is ideal for OLTP databases but does not perform well in an analytical workload.
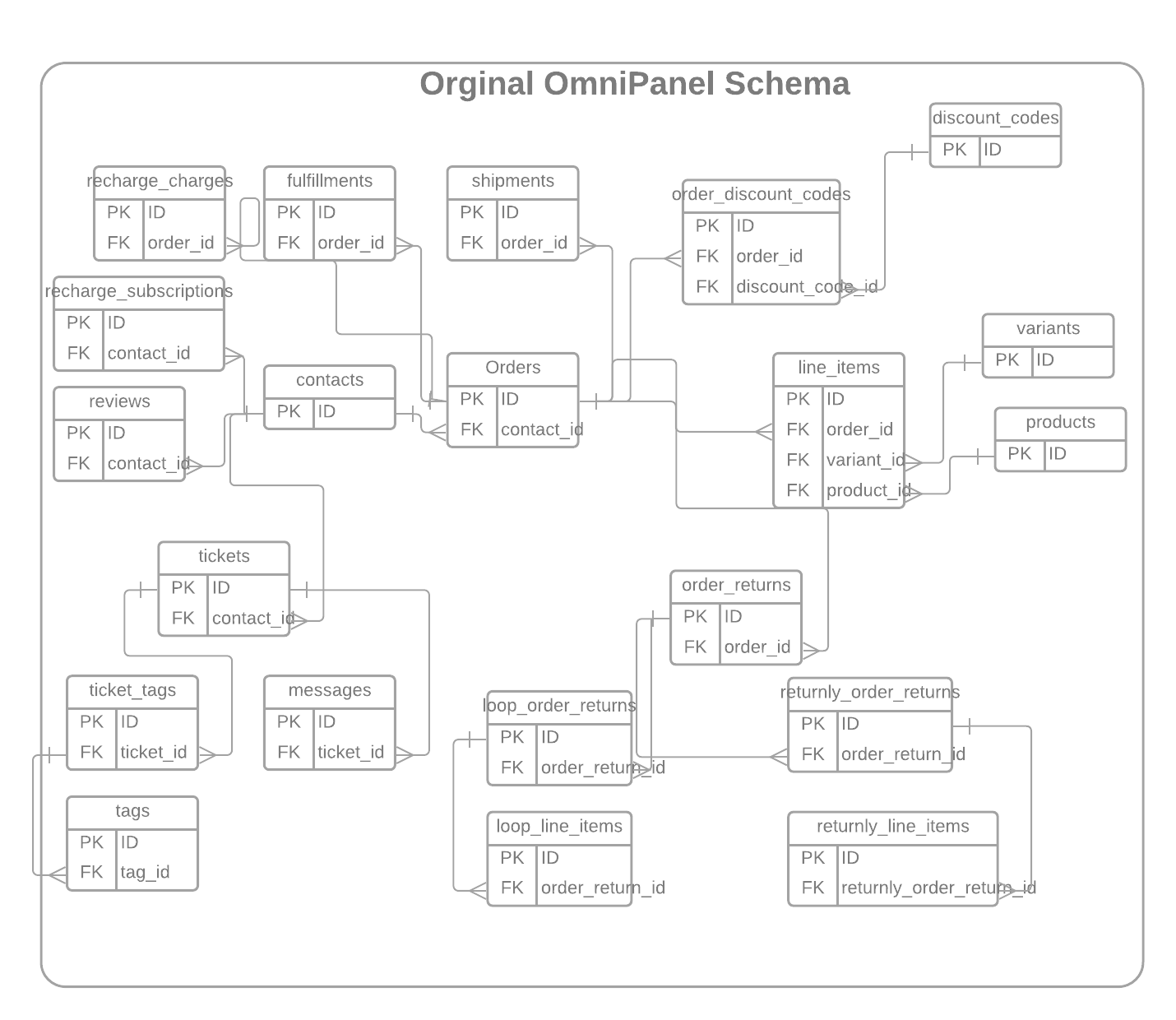
Within Firebolt we denormalized the data and created a data model which was far more efficient for analytical workload.

Architecture

Dashboards
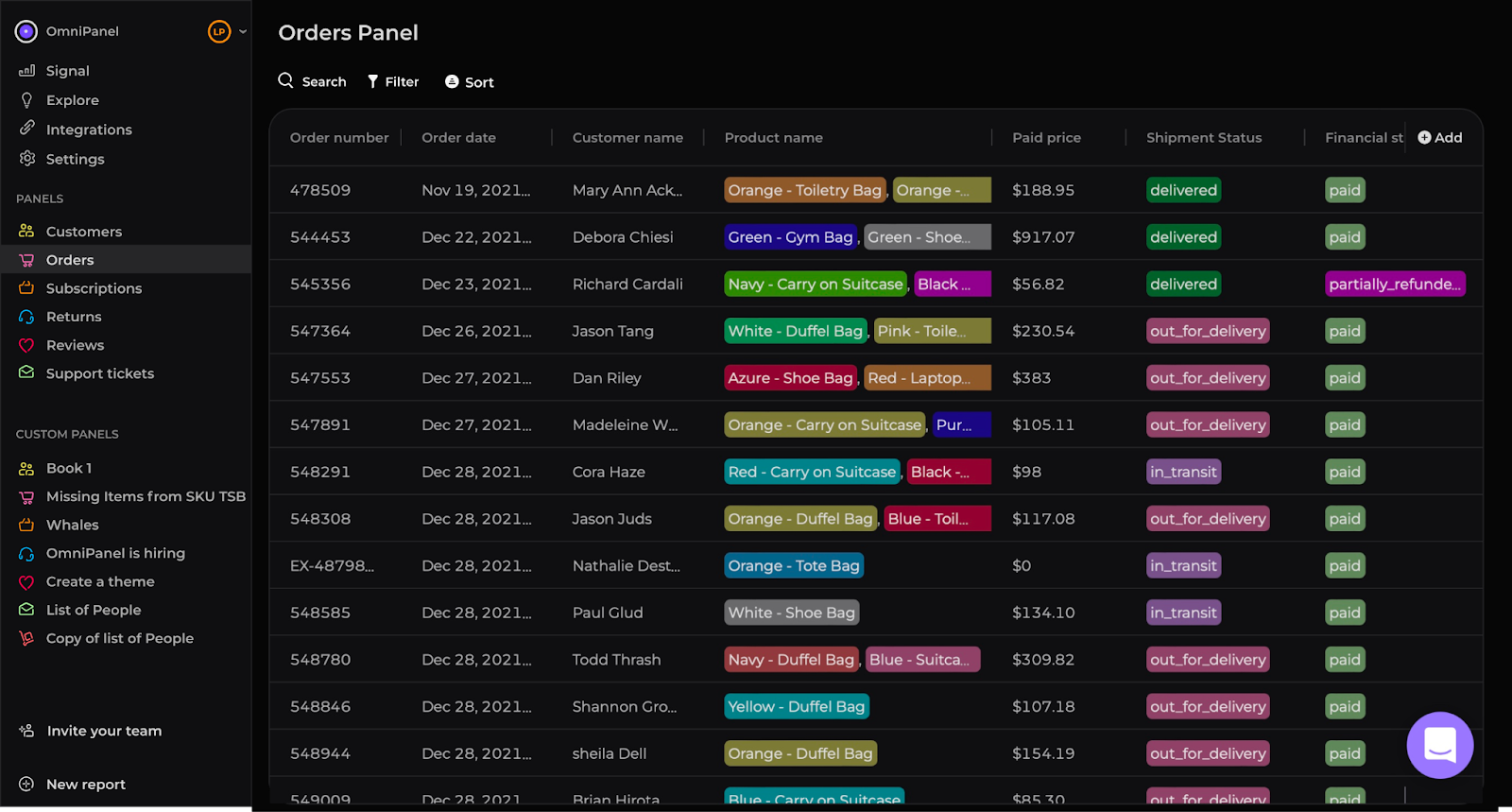
Firebolt allowed us to build the product we always envisioned building, fully accompanied with robust features. Firebolt opened up the aperture of our product's desired features and capabilities.
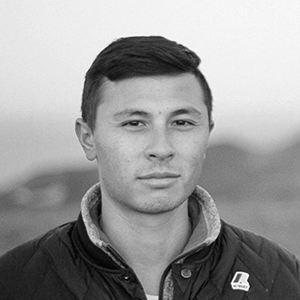