Listen to this article
TL;DR: Legacy BI tools can’t keep up with AI. Firebolt Forward revealed 5 trends defining the future:
- AI-first infra: Sub-second queries, high concurrency, vector support
- Unified platforms: One system for all workloads
- Flexible deployment: Self-hosted or cloud, your choice
- Lakehouse adoption: Open formats + warehouse performance
- AI-powered ops: From text-to-SQL to smart tuning
The recent Firebolt Forward event highlighted a critical challenge facing data teams today: we're building infrastructure for the AI revolution using tools designed for the BI dashboard era. This fundamental disconnect is causing significant pain points - from performance bottlenecks to cost overruns - as organizations struggle to adapt legacy systems to new AI-driven workloads.
You can check out the event here: Firebolt Forward: Data Warehousing in the Age of AI
Based on the presentations and customer testimonials from the event, here are five key trends shaping the future of data warehousing in the AI era:
1. AI Applications Demand a New Infrastructure
Traditional data warehouses were built for business intelligence dashboards where acceptable performance meant reports loading in under 10 seconds. Today's AI applications have dramatically different requirements:
- Sub-second query responses: AI applications need millisecond-level latency
- Ultra-high concurrency: Supporting thousands of simultaneous users
- Cost efficiency at scale: Maintaining performance without breaking the bank
As Igor Stanko, Firebolt's Chief Product Officer, explained:
"Traditional data warehouses were built for BI dashboards where acceptable performance meant bar charts rendering in under 10 seconds. Today's AI applications demand responses in milliseconds, support for thousands of concurrent users, and seamless handling of both structured and unstructured data including vectors and embeddings."
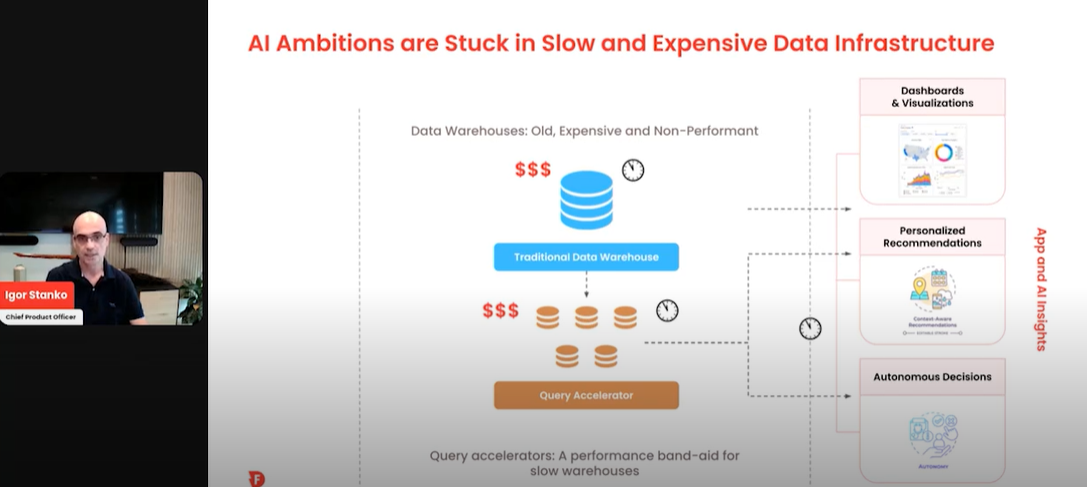
According to research from ISG presented by David Menninger, Executive Director at ISG:
"The data shows that 80% of AI project time is spent wrangling data, not building models. And 35% of enterprises cite poor infrastructure as their biggest blocker to generative AI adoption."
2. The Move Toward Unified Data Platforms
Organizations are tired of maintaining specialized systems for each workload type - one system for BI, another for real-time analytics, a separate vector database for semantic search, and so on. The trend is toward unified platforms that can handle diverse workloads within a single architecture.
As Igor Stanko outlined:
"Fireball value proposition extends to supporting high speed data ingestion, which ensures rapid ingestion and availability of both structure as well as semi structured data sets to feed those air systems efficient data processing to support the preparation of clean data sets, regardless of the data size or complexity."
3. Self-Hosted Flexibility Alongside Cloud Convenience
While cloud-native architectures remain dominant, organizations increasingly want deployment flexibility without sacrificing performance. Benjamin Wagner, VP of Engineering at Firebolt, emphasized this point when introducing Firebolt Core:
"If you don't want to use Firebolt as a fully managed service, we are now also launching our Firebolt core offering. Firebolt core is free to use and self-hosted, and you can really deploy it wherever you want. You can run it on your own laptop, you can run it on AWS, you can run it on GCP. You can even run it in your data center, if that's what you want."
4. The Convergence of Data Warehouse and Data Lake
The artificial separation between data warehouses and data lakes is dissolving. Modern platforms are adopting a "lakehouse" approach where they can work directly with open formats like Apache Iceberg while still providing warehouse-level performance.
Igor Stanko noted:
"Many organizations today are adopting Lake houses and open file formats with the promise of having a unified data layer that supports various purpose built query engines that sit on top. Main value proposition being to avoid complex and costly data movement operations across various data stores. We at Firebolt are seeing lots of organizations adopting iceberg as a core technology and open file format to support those Lake House deployments."
5. AI-Enhancement of Data Infrastructure Itself
Perhaps the most meta trend is how AI is being used to improve data infrastructure management. Hiren Patel, Head of Product at Firebolt, outlined this vision:
"Our focus here are in two key areas, enhancing the end user experience using AI, where we are building AI specific Q and A chatbot for Firebolt itself, enabling users to develop their applications from text to SQL, providing AI driven workload optimization tool such as primary and aggregated index recommendation and AI power migrations from other platform to Firebolt."
Real-World Impact
The customer stories presented at Firebolt Forward validated these trends. Ryan McWilliams, CTO of Vrio, shared:
"Before Firebolt, we were paying astronomical costs to AWS and trying to solve our issues with MySQL and RDS and Aurora, and nothing would work. It was probably every day I'd be pinged with somebody saying a report's taking too long, or it's not completing at all."
After migrating to Firebolt:
"We probably save 50% of the money that we are spending on AWS and RDS and other solutions for analytics. We moved to Firebolt just because the way that it runs, the way that it's set up, it's fast, it's available, and we can always count on it."
Yan Cohen from Bigabid described similar transformative results:
"Our campaign managers always wanted more and more, and a lot of times things got stuck, or we had to do trade-offs between analyzing a couple of months or seeing the entire data funnel."
With Firebolt:
"Our ingestion is real near time, and it really helps our campaign managers understanding if they have an issue with the campaign... If they have an issue, where they can promote additional campaigns."
The Bottom Line
The data warehouse of the AI era looks fundamentally different from its predecessors. It's not just about storage capacity or SQL compatibility - it's about millisecond-level performance at scale, seamless handling of diverse data types (including vectors), and the flexibility to deploy anywhere while maintaining a unified architecture.
As organizations continue to build and deploy AI-powered applications, this evolution of data infrastructure will be a critical factor in their success. The companies that adapt quickest to these new requirements will have a significant competitive advantage in the AI-driven future.